The Ultimate Guide to Data Labeling for Machine Learning
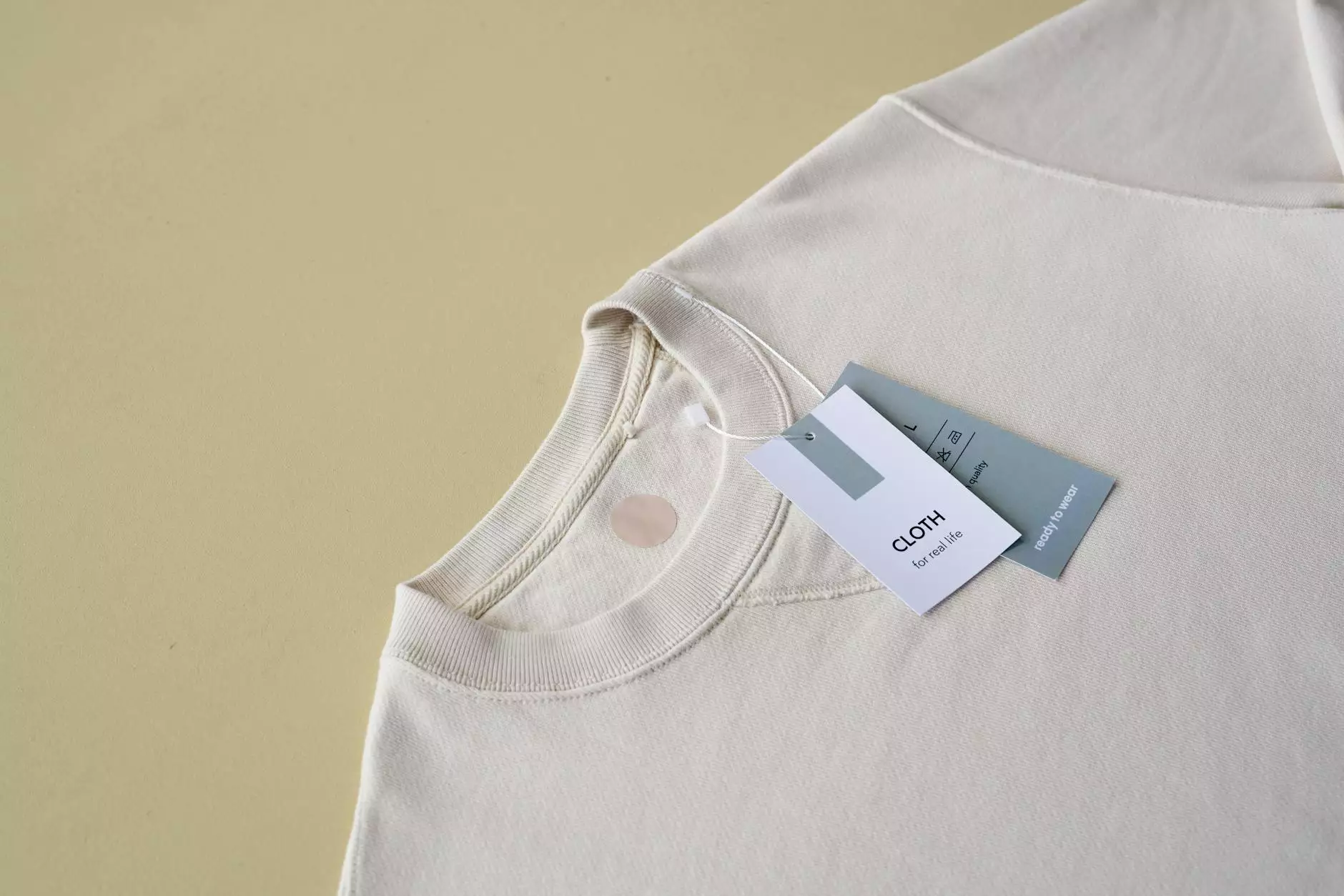
In the rapidly evolving field of machine learning, data labeling has emerged as a critical component that can significantly impact the performance of machine learning models. This article delves deep into the world of data labeling, exploring its importance, methods, tools, and how platforms like KeyLabs.ai are defining the future of data annotation.
Understanding Data Labeling in Machine Learning
At its core, data labeling refers to the process of annotating or tagging datasets so that machines can learn from them. This process is essential for supervised learning algorithms, which rely on labeled data to make predictions or decisions based on unseen datasets. Without accurate labels, machine learning models cannot learn effectively, leading to poor performance and unreliable results.
The Importance of Data Labeling
The significance of data labeling cannot be overstated. Here are some of the main reasons why it is crucial for machine learning:
- Improves Model Accuracy: High-quality labeled data directly correlates with the accuracy of machine learning models. Accurate labels provide a clear learning path for the algorithms.
- Facilitates Data Understanding: Labeling data helps in contextualizing information, making it easier for data scientists to extract insights and trends.
- Enhances Decision-Making: Well-labeled data informs business decisions, contributing to better strategies and outcomes.
- Ensures Compliance: In industries like healthcare and finance, accurate data labeling is essential for adherence to regulations and ensuring consumer protection.
Types of Data Labeling
Data labeling can be categorized into multiple types, each suited for different applications within machine learning. Understanding these types can help businesses choose the right approach for their needs:
1. Image Annotation
Image annotation involves labeling images with relevant tags or bounding boxes to identify objects, actions, or features. This type of labeling is essential in computer vision tasks such as face recognition, object detection, and image segmentation.
2. Text Annotation
This encompasses various tasks such as entity recognition, sentiment analysis, and language translation. Text annotation is vital for applications like natural language processing (NLP) and chatbots.
3. Video Annotation
Video annotation is used for tasks that involve temporal data, such as activity recognition or object tracking in videos. This is essential for applications in autonomous driving and security surveillance.
4. Audio Annotation
Audio annotation includes labeling sounds, identifying speakers, or transcribing speech. This is particularly useful in speech recognition systems and virtual assistants.
Data Annotation Techniques
There are various techniques employed in data annotation, each with its strengths and limitations. Here’s a closer look at some of the most common methods:
- Manual Annotation: This method involves human annotators labeling data based on predefined guidelines. While it can be time-consuming and prone to human error, it often yields the best quality results.
- Automated Annotation: Leveraging algorithms to pre-label data can save time and reduce costs. However, the effectiveness largely depends on the complexity of the data and the sophistication of the algorithms used.
- Crowdsourcing: Utilizing platforms to gather labels from a large number of people can be a cost-effective way to annotate data quickly. However, maintaining quality control becomes crucial in this method.
- Active Learning: This machine learning technique involves iteratively training a model and only labeling those data points that the model is least confident about, optimizing the labeling process for efficiency.
Challenges in Data Labeling
Despite its importance, data labeling comes with challenges. Here are some of the key obstacles faced by businesses:
1. Scale and Volume
The sheer volume of data generated today is monumental. Managing this data and labeling it effectively can overwhelm resources.
2. Quality Control
Ensuring the quality of labeled data is paramount. Incorrect labels can mislead algorithms, leading to subpar model performance.
3. Domain-Specific Knowledge
Some datasets require specialized knowledge for accurate labeling. For instance, labeling medical images requires expertise that not every annotator possesses.
4. Cost Implications
High-quality labeling, especially manual, can be expensive. Finding a balance between speed, cost, and quality is a common challenge.
The Role of KeyLabs.ai in Data Annotation
As businesses seek to enhance their machine learning capabilities, partnering with experts in data annotation becomes critical. KeyLabs.ai stands at the forefront of this field, offering cutting-edge solutions for data labeling. Here’s how they contribute:
1. Advanced Annotation Tools
KeyLabs.ai provides comprehensive data annotation tools that streamline the process, allowing for efficient and effective data labeling. Their platform supports multiple data types, from images to texts, ensuring flexibility across various domains.
2. Expert Team
With a team of skilled annotators who understand the intricacies of data labeling, KeyLabs.ai ensures high-quality annotations that enhance machine learning outcomes.
3. Quality Assurance Processes
The platform implements rigorous quality control measures to ensure that the labeled data meets industry standards. This involves periodic reviews and feedback loops to maintain high accuracy.
4. Scalable Solutions
KeyLabs.ai understands the scalability challenges faced by businesses. Their solutions are designed to scale with your project, accommodating growing datasets without compromising quality.
Best Practices for Data Labeling
For organizations looking to optimize their data labeling processes, adhering to best practices is essential. Here are some recommendations:
- Define Clear Guidelines: Establish specific labeling guidelines to ensure consistency across annotators.
- Invest in Training: Provide comprehensive training for annotators, especially for complex datasets that require specialized knowledge.
- Utilize Feedback Mechanisms: Implement feedback systems to refine the labeling process continuously and address any discrepancies.
- Leverage Technology: Use annotation tools that incorporate machine learning to facilitate and expedite the labeling process.
- Evaluate Quality Regularly: Regular assessments of the labeled data help in identifying issues and ensuring high-quality outputs.
Conclusion
In conclusion, effective data labeling for machine learning is a foundational step that can greatly influence the success of AI and machine learning projects. With platforms like KeyLabs.ai providing robust data annotation tools and expert services, businesses can overcome the challenges of data labeling and enhance their machine learning initiatives. As the demand for quality labeled data continues to grow, investing in a reliable data annotation platform will be key to staying competitive in the fast-paced digital landscape.
data labeling machine learning