Unlocking the Power of Data Labelling Tools for Your Business
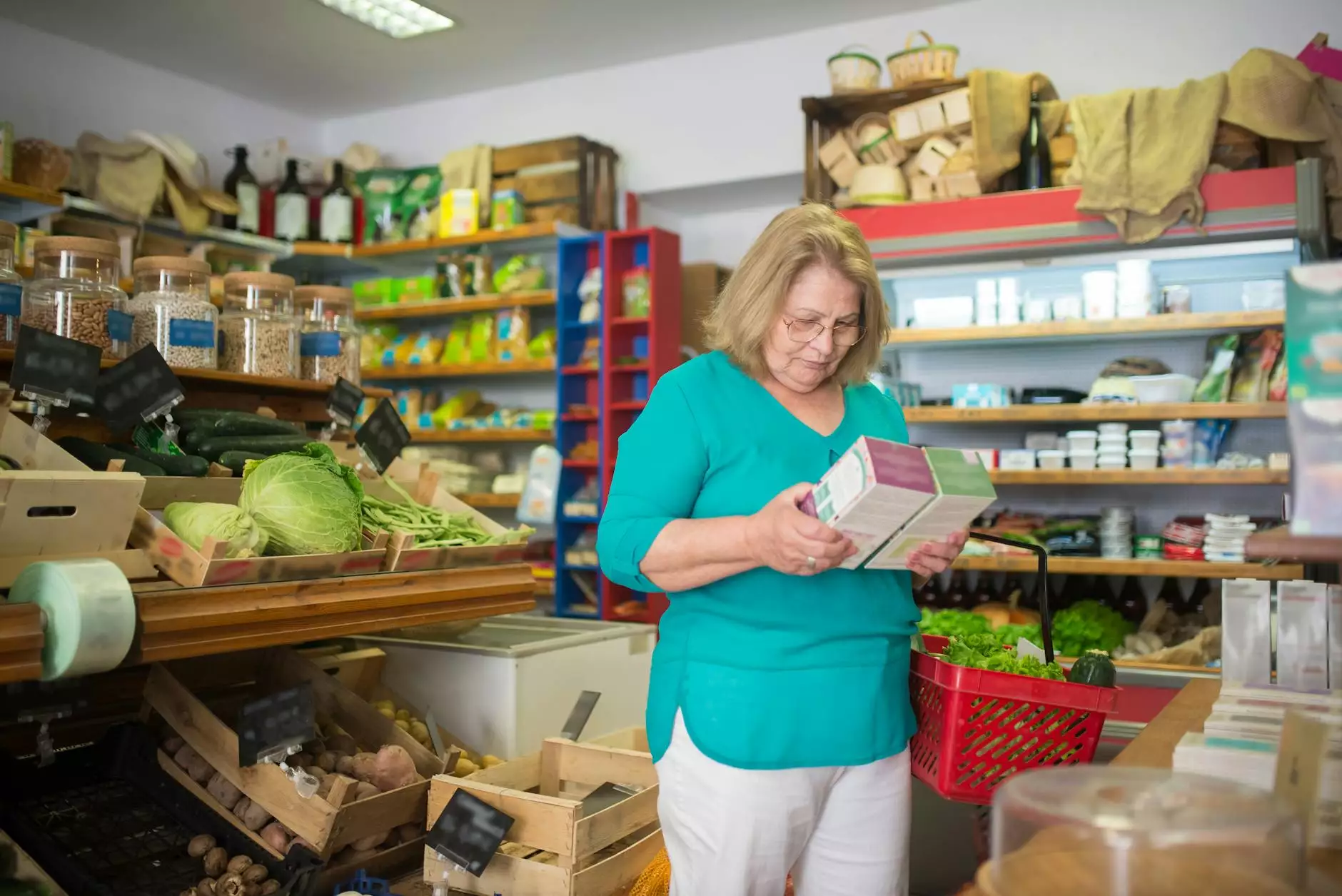
In the ever-evolving landscape of artificial intelligence and machine learning, the importance of high-quality data cannot be overstated. Businesses today are leveraging advanced data labelling tools to classify, annotate, and prepare their datasets for a variety of applications, from image recognition to natural language processing. This article dives deep into the world of data labelling, exploring its significance, benefits, and how it can dramatically enhance your business processes.
Understanding Data Labelling Tools
Data labelling tools are software solutions designed to assist businesses in the annotation of data for training machine learning models. They provide an interface for users to easily classify and tag data points, ensuring that machines learn from accurately labelled datasets. This process is crucial, as the performance of AI models heavily relies on the quality of the training data used.
Types of Data Labelling Tools
There are various types of data labelling tools, classified based on their functionality and the types of data they handle:
- Image Annotation Tools: Used for labelling images by drawing bounding boxes, polygons, or key points.
- Text Annotation Tools: Suitable for annotating text data, such as sentiment analysis, named entity recognition, and more.
- Audio Annotation Tools: Designed for annotating audio datasets, including speech recognition tasks and sound classification.
- Video Annotation Tools: Used for labelling video data, important for applications in surveillance, autonomous vehicles, and more.
The Importance of Data Labelling in AI Today
The realm of artificial intelligence is profoundly reliant on data. The models that drive innovation require vast amounts of accurately labelled data. Here's why using a capable data labelling tool is essential for any business looking to implement AI technologies:
1. Enhancing Data Quality
A high-quality dataset directly correlates with the performance of machine learning models. Unlabelled or poorly labelled data can introduce biases and inaccuracies, leading to subpar performance. Effective data labelling tools help maintain data integrity.
2. Boosting Model Accuracy
Accurate labels allow models to learn patterns effectively. Using a sophisticated data labelling platform, businesses can ensure that their models receive the most informative feedback, which is essential for machine learning success.
3. Accelerating Project Timelines
Manually labelling data can be a tedious and time-consuming process. Implementing efficient data labelling tools can significantly reduce the time spent on data preparation, allowing teams to focus on developing and deploying models faster.
4. Supporting Collaboration
Modern data annotation platforms enable team collaboration, where multiple users can contribute to the labelling process. This feature is particularly beneficial for large-scale projects needing input from various experts across different domains.
Key Features to Look for in Data Labelling Tools
When selecting a data labelling tool for your business, it’s important to consider several key features that can greatly impact your workflow:
- User-Friendly Interface: An intuitive design can streamline the labelling process, making it easier for users to navigate and complete tasks.
- Flexibility: The tool should support a variety of data formats, including images, videos, texts, and audio.
- Automation Capabilities: Look for tools that offer automation in labelling tasks through features such as pre-labelling or machine learning-assisted annotation.
- Integration with Other Tools: Ensure that your selected tool can seamlessly integrate with other software used in your data pipeline.
- Robust Quality Control: Effective quality assurance features that allow you to review and rectify labelled data significantly enhance the dataset's overall quality.
The Process of Data Labelling
Here’s a comprehensive look at the typical process involved in data labelling using specialized tools:
1. Data Collection
The first step involves gathering data from various sources such as databases, APIs, or web scraping tools. This data might be unlabelled and will require annotation.
2. Data Preparation
Before labelling, the data may need preprocessing to clean and format it appropriately. This step ensures that the data is ready for annotation.
3. Annotation
Using the data labelling tool, users will tag and classify the data. This can involve drawing bounding boxes around objects in images, highlighting relevant phrases in text, or segmenting parts of an audio file.
4. Quality Assurance
After the annotation is complete, a quality assurance process is essential. This may involve reviewing the labelled data for accuracy and consistency. Many tools offer options for peer review within the platform.
5. Exporting Data
Once quality checks are satisfactory, the final annotated data can be exported in various formats for training machine learning models.
Applications of Data Labelling Tools
The versatility of data labelling tools means they are applicable across numerous industries. Here are a few notable examples:
1. Healthcare
In the healthcare industry, data labelling is crucial for developing diagnostic models. For instance, labelling medical images can aid in training AI to identify tumors or other conditions.
2. Autonomous Vehicles
Autonomous vehicles rely on accurate data labelling for sensor data, including images from cameras and radar signals. Properly labelled datasets enable these vehicles to recognize road signs, pedestrians, and potential hazards.
3. E-commerce
E-commerce platforms use data labelling to enhance product recommendations. By annotating user data and preferences, businesses can personalize shopping experiences, ultimately driving sales.
4. Natural Language Processing
For systems focused on language understanding, text annotation helps in sentiment analysis, intent recognition, and chatbot training.
Choosing the Right Data Labelling Solution
With numerous options available, selecting the right data labelling tool can be challenging. Here are some steps to guide you:
1. Define Your Needs
Identify the specific requirements of your project. Understanding what data you will be labelling and the output you expect will help narrow down your options.
2. Evaluate Available Tools
Research different tools available in the market. Look for reviews, case studies, and feature comparisons.
3. Trial Versions
Many tools offer trial versions. Take advantage of these to explore user experience and determine whether it meets your needs.
4. Support and Community
Check for customer support availability and whether there is an active community. A strong community can be a valuable resource for troubleshooting and tips.
Conclusion: The Future of Data Labelling Tools
As businesses increasingly rely on artificial intelligence to drive their operations, the importance of efficient and accurate data labelling will continue to grow. Investing in a top-notch data labelling tool is not just a choice but a necessity for scalable AI applications.
By enhancing data quality, improving model accuracy, and accelerating project timelines, these tools become invaluable assets in the pursuit of operating excellence. For businesses serious about leveraging AI, the journey begins with understanding the significance of data labelling and making the right choices for their data management strategies.
Explore more about our solutions at keylabs.ai and discover how our state-of-the-art data labelling tools can transform your business's approach to AI!